When Algorithms Compete, Who Wins?
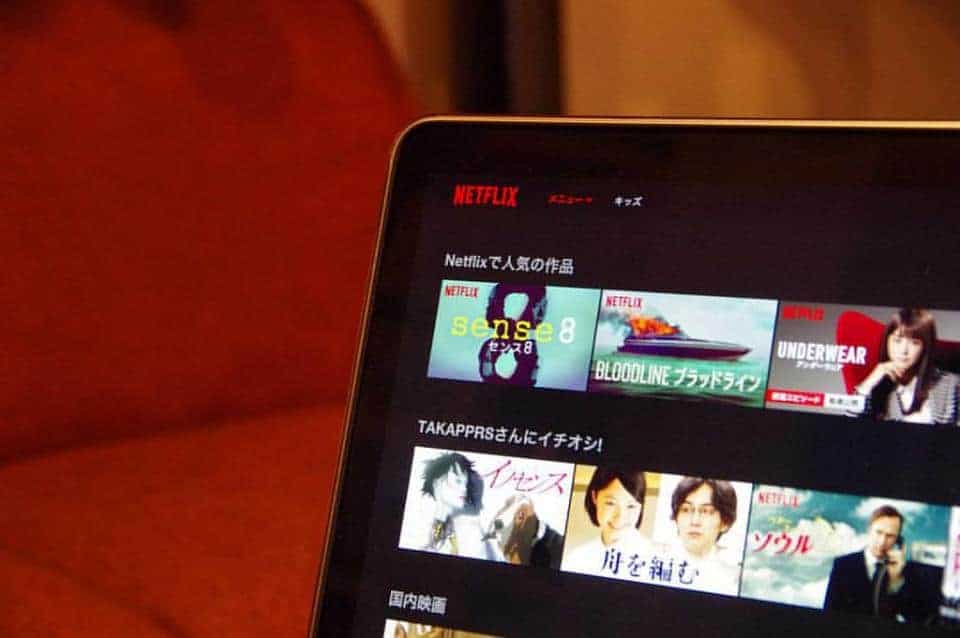
Companies like Netflix and Hulu compete for subscribers to make sure their businesses thrive. But there’s another type of competition at work that receives far less attention – the competition among the machine learning algorithms used by these kinds of competitor companies.
James Zou, Stanford assistant professor of biomedical data science and an affiliated faculty member of the Stanford Institute for Human-Centered Artificial Intelligence, says that as algorithms compete for clicks and the associated user data, they become more specialized for subpopulations that gravitate to their sites. And that, he finds in a new paper with graduate student Antonio Ginart and undergraduate Eva Zhang, can have serious implications for both companies and consumers.
Perhaps consumers don’t mind if Hulu recommendations seem intended for urban teenagers or Netflix offers better choices for middle-aged rural men, but when it comes to predicting who should receive a bank loan or whose resume should reach a hiring manager, these algorithms have real-world repercussions.
“The key insight is that this happens not because the businesses are choosing to specialize for a specific age group or demographic,” Ginart says. “This happens because of the feedback dynamics of the competition.”
Inevitable Specialization
Before they started their research, Zou’s team recognized that there’s a feedback dynamic at play if companies’ machine learning algorithms are competing for users or customers and at the same time using customer data to train their model. “By winning customers, they’re getting a new set of data from those customers, and then by updating their models on this new set of data, they’re actually then changing the model and biasing it toward the new customers they’ve won over,” Ginart says.
The team wondered: How might that feedback affect the algorithms’ ability to provide quality recommendations? To get at an answer, they analyzed algorithmic competition mathematically and simulated it using some standard datasets. In the end, they found that when machine learning algorithms compete, they eventually (and inevitably) specialize, becoming better at predicting the preferences of a subpopulation of users.
“It doesn’t matter how much data you have, you will always see these effects,” Zou says. Moreover, “The disparity gets larger and larger over time – it gets amplified because of the feedback loops.”
In addition, the team showed that beyond a certain mathematically calculable number of competitors, the quality of predictions declines for the general population. “There’s actually a sweet spot – an optimal number of competitors that optimizes the user experience,” Ginart says. Beyond that number, each AI agent has access to data from a smaller fraction of users, reducing their ability to generate quality predictions.
The team’s mathematical theorems apply whenever an online digital platform is competing to provide users with predictions, Ginart says. Examples in the real world include companies that use machine learning to predict users’ entertainment preferences (Netflix, Hulu, Amazon) or restaurant tastes (Yelp, TripAdvisor), as well as companies that specialize in search, such as Google, Bing, and DuckDuckGo.
“If we go to Google or Bing and type in a search query, you could say that what Google is trying to do is predict what links we will consider most relevant,” Ginart says. And if Bing does a better job of making those predictions, maybe we’ll be more inclined to use that platform, which in turn alters the input into that machine learning system and changes the way it makes predictions in the future.
The theorems also apply to companies that predict users’ credit risk or even the likelihood that they will jump bail. For example, a bank may become very good at predicting the creditworthiness of a very specific cohort of people – say, people over the age of 45 or people of a specific income bracket – simply because they’ve gathered a lot of data for that cohort. “The more data they have for that cohort, the better they can service them,” Ginart says. And although these algorithms get better at making accurate predictions for one subpopulation, the average quality of service actually declines as their predictions for other groups become less and less accurate.
Imagine a bank loan algorithm that relies on data from white, middle-aged customers and therefore becomes adept at predicting which members of that population should receive loans. That company is actually missing an opportunity to accurately identify members of other groups (Latinx millennials, for example) who would also be a good credit risk. That failure, in turn, sends those customers elsewhere, reinforcing the algorithm’s data specialization, not to mention compounding structural inequality.
Seeking Solutions
In terms of next steps, the team is looking at the effect that buying datasets (rather than collecting data only from customers) might have on algorithmic competition. Zou is also interested in identifying some prescriptive solutions that his team can recommend to policymakers or individual companies. “What do we do to reduce these kinds of biases now that we have identified the problem?” he says.
“This is still very new and quite cutting-edge work,” Zou says. “I hope this paper sparks researchers to study competition between AI algorithms, as well as the social impact of that competition.”
Stanford HAI’s mission is to advance AI research, education, policy and practice to improve the human condition. Learn more.
from ScienceBlog.com https://ift.tt/3kK2JMl
Comments
Post a Comment